NVIDIA has made a significant leap in the field of artificial intelligence with the release of Nemotron-4 340B, a comprehensive suite of open models designed to generate synthetic data for training large language models (LLMs). This innovative family of models aims to democratize access to high-quality training data, which is crucial for developing accurate and effective LLMs, especially in commercial applications spanning industries such as healthcare, finance, manufacturing, and retail.
Addressing the Data Challenge
High-quality training data is the backbone of any successful LLM. It ensures the model’s performance, accuracy, and the quality of responses. However, obtaining robust datasets can be prohibitively expensive and challenging. This is where Nemotron-4 340B steps in, offering a scalable, cost-effective solution. Through its permissive open model license, developers can freely generate synthetic data, making it easier to build powerful LLMs without the heavy burden of data acquisition costs.
The Nemotron-4 340B Family
The Nemotron-4 340B suite includes three core models: base, instruct, and reward. Together, they create a pipeline that facilitates the generation and refinement of synthetic data. These models are optimized to work seamlessly with NVIDIA NeMo, an open-source framework that supports end-to-end model training, including data curation, customization, and evaluation. Additionally, they are tailored for efficient inference using the NVIDIA TensorRT-LLM library.
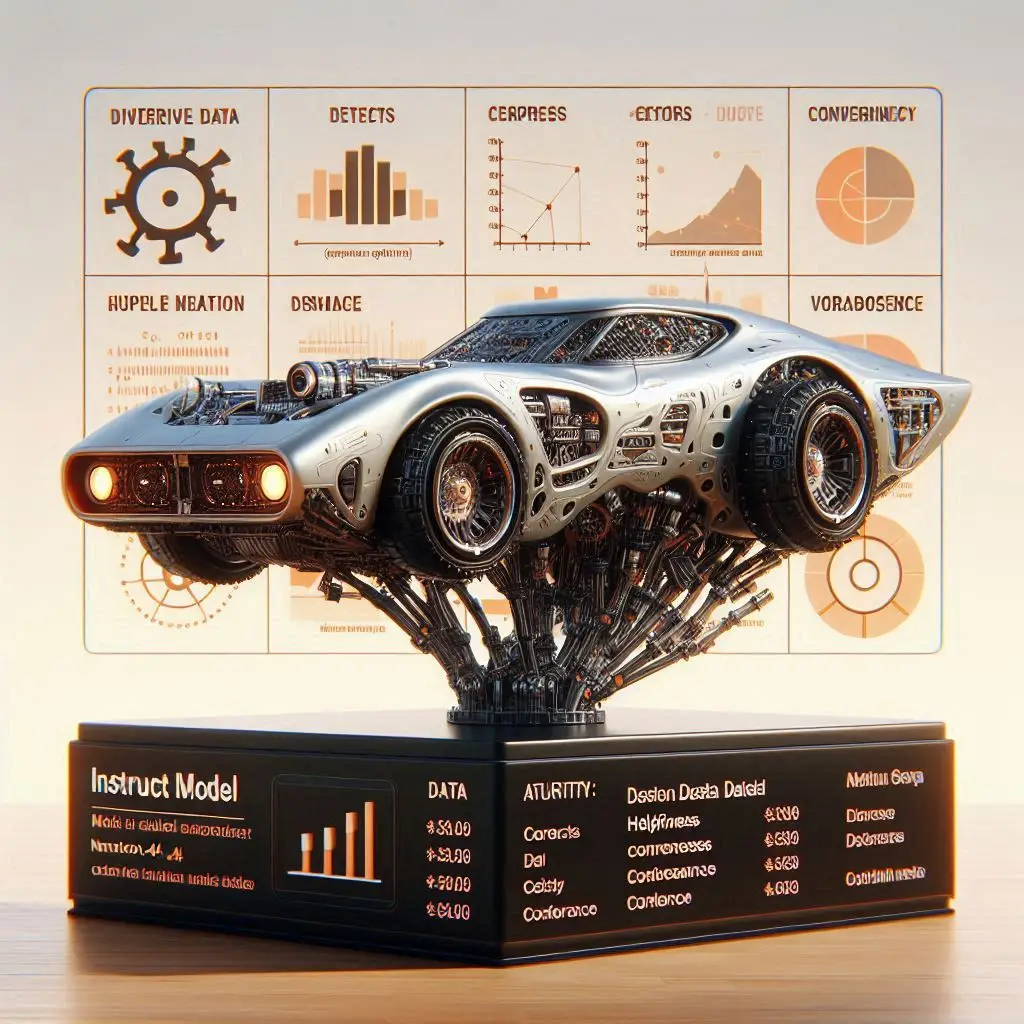
Nemotron-4 340B Instruct Model: This model generates diverse synthetic data that emulates real-world data, improving data quality and enhancing the performance of custom LLMs across various domains
Nemotron-4 340B Reward Model: To further refine the quality of AI-generated data, this model filters for high-quality responses, evaluating them based on attributes such as helpfulness, correctness, coherence, complexity, and verbosity. It currently holds the top position on the Hugging Face RewardBench leaderboard, underscoring its effectiveness in ensuring high standards of generated data.
The Synthetic Data Generation Pipeline
The pipeline begins with the Nemotron-4 340B Instruct model, which produces synthetic text-based output. This output is then evaluated by the Nemotron-4 340B Reward model, which provides feedback to guide iterative improvements. This process ensures that the synthetic data generated is accurate, relevant, and aligned with specific requirements.
Developers can further customize the Nemotron-4 340B Base model using proprietary data and the included HelpSteer2 dataset. This customization allows for the creation of tailored instruct or reward models that meet specific domain needs.
Optimizing with NeMo and TensorRT-LLM
Leveraging the open-source NVIDIA NeMo and TensorRT-LLM, developers can enhance the efficiency of their models. Nemotron-4 340B models are optimized with TensorRT-LLM to utilize tensor parallelism, which splits individual weight matrices across multiple GPUs and servers. This enables efficient inference at scale, a critical capability for handling large-scale synthetic data generation tasks.
The Nemotron-4 340B Base model, trained on 9 trillion tokens, can be fine-tuned using the NeMo framework to adapt to particular use cases. Various customization methods, including supervised fine-tuning and low-rank adaptation (LoRA), are available, enabling more precise outputs for specific downstream tasks.
Ensuring Quality and Safety
Alignment is a crucial step in training LLMs. With NeMo Aligner and datasets annotated by Nemotron-4 340B Reward, developers can align their models to ensure safe, accurate, and contextually appropriate outputs. The alignment process often involves reinforcement learning from human feedback (RLHF), which further refines the model’s behavior to meet intended goals.
Enterprise-Grade Support
For businesses requiring robust support and security, NVIDIA offers NeMo and TensorRT-LLM through the NVIDIA AI Enterprise software platform. This cloud-native platform provides accelerated and efficient runtimes for generative AI foundation models, ensuring enterprise-grade reliability for production environments.
Conclusion
NVIDIA’s release of Nemotron-4 340B marks a pivotal advancement in the realm of synthetic data generation for training LLMs. By providing an open, scalable solution, NVIDIA is empowering developers to overcome the significant challenge of acquiring high-quality training data. This innovation not only enhances the development of custom LLMs but also democratizes access to AI advancements across various industries. With the integration of Nemotron-4 340B models into NVIDIA’s ecosystem, developers are equipped with the tools needed to generate and refine synthetic data, ensuring their models achieve the highest standards of performance and accuracy.
Add a Comment: